December 03, 2020 | News | Interview
Where Social Science meets Computational Science
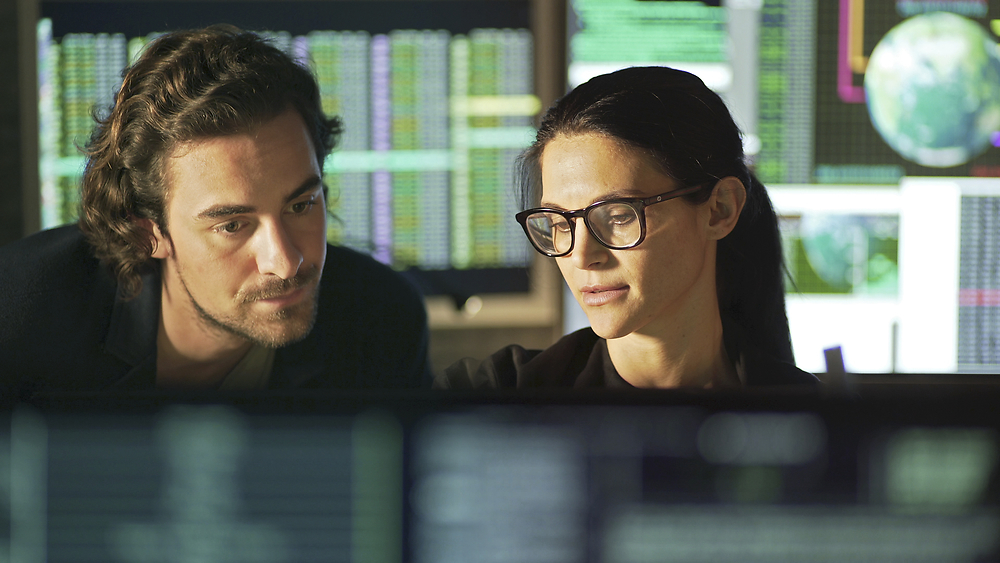
© iStockphoto.com/Laurence Dutton
A new field of research has emerged. Computational Social Science is an interdisciplinary area combining computing and social sciences. Demography is at the very heart of it. In this interview, Samin Aref, computer scientist at MPIDR, introduces us to the new field.
Dr. Aref, how would you define Computational Social Science in one sentence?
For me, Computational Social Science, or CSS, is like pieces of a puzzle coming together to form a new scientific field and accelerate scientific discovery.
What exactly does that mean?
Recent scientific trends have led researchers from historically separate disciplines to come together and create an inclusive and welcoming community that combines computational sciences and social sciences to find a new path of empirical research and scientific theory.
Why is CSS gaining momentum among a really diverse group of researchers right now?
One reason is the Information Revolution. The amount of new information produced every two years is now more than all the information produced ever before. This exponential overflow of information, which is almost entirely digital, has led many researchers to think differently about data, and particularly data for research.
But how these trends in digital data and information are related to social science and humanities?
A substantial amount of these novel data are related to human activity and social interactions. They are passively collected as the by-product of the digital technologies we interact with. These footprints we leave behind in the digital world are considered big data; differing in volume, velocity, variety, and veracity from the research data collected using traditional methods.
So, establishing a new field of research is kind of a natural adaptation to the overflow of big social data?
Exactly! Besides these trends, the exponential increase in the computational power and the new computing capabilities made possible by Artificial Intelligence correspond to the requirements of the 21st century data-rich social science. Artificial Intelligence is already a part of our social environment in many ways and impacts our most fundamental social decisions: for example, an increasing number of relationships and marriages are initiated through the use of dating apps and algorithmic matchmaking.
Sounds like this comes along with opportunities and challenges…
Yes, it raises new ethical, legal, and social concerns on privacy, data infrastructure, and access. We see a delay in understanding the societal impacts of the digital life in research compared to the speed of technological progress. Policymakers also have a hard time keeping up with regulating the collection, access, sharing, and the use of digital data. The disparities between academia and the industry in access to big social data and skills for making sense of them are creating a digital divide which may have undesirable consequences at the societal level.
What can be done about it?
Many scientists who are not necessarily experts in computing, privacy, or information science recognize these trends, acknowledge these concerns, and appreciate their relevance to social sciences. They consider the necessity of this interdisciplinary field for solving societal challenges through the synergy of conducing social and computational research collaboratively. Cross-disciplinary training initiatives are essential for reducing the digital divide. As a part of an online course at the MPIDR, I recently had the opportunity to teach big data and computational social science. 129 students from 43 countries with a wide range of disciplinary backgrounds were taking part. I see recent developments in CSS as ways of not standing on the sidelines or leaving the analysis of big social data to tech giants and governments. CSS can help prevent a new age of data scandals, privacy invasions, and AI-enabled human rights suppression.
With the MPIDR’s focus on demography, what is the role of demographic research in CSS?
As a newcomer and certainly a non-expert, I see demography as the quantitative and observational subfield of social sciences which has benefited from inclusive and cross-disciplinary methods and approaches to making sense of data for many decades. Demography has a very special role in CSS offering key procedural knowledge in combining and analyzing large-scale data from heterogenous sources. It is no surprise that we do not hear the term “big data” among demographers as much as we may hear it in other scientific communities. For demographers, data have always been big in a sense.
About
Before Samin Aref joined the Max Planck Institute for Demographic Research (MPIDR) in 2018, he was a computer science PhD student at the University of Auckland. There he was working on developing computational methods and high-performance algorithms for a theoretical research question coming from social science: whether the enemy of an enemy is a friend. He was affiliated with the Center for Mathematical Social Science and Te Pūnaha Matatini which supported him in conducting cross-disciplinary research.
At the MPIDR Samin Aref works closely with his fellow colleagues who are trained in demography or in related social science disciplines to address pressing questions in key areas of population science, including migration and mobility, aging and generational processes, and social and environmental dynamics. Building on solid methodological foundations, they use a combination of traditional and unconventional data sources, as well as novel computational approaches.
References
- boyd, danah, & Crawford, K. (2012). Critical Questions for Big Data. Information, Communication & Society, 15(5), 662–679. DOI: 10.1080/1369118X.2012.678878
- Cesare, N., Lee, H., McCormick, T., Spiro, E., & Zagheni, E. (2018). Promises and Pitfalls of Using Digital Traces for Demographic Research. Demography, 55(5), 1979–1999. DOI: 10.1007/s13524-018-0715-2
- Edelmann, A., Wolff, T., Montagne, D., & Bail, C. A. (2020). Computational Social Science and Sociology. Annual Review of Sociology, 46(1), 61–81. DOI: 10.1146/annurev-soc-121919-054621
- Hilbert, M., & López, P. (2011). The World’s Technological Capacity to Store, Communicate, and Compute Information. Science, 332(6025), 60–65. DOI: 10.1126/science.1200970
- King, G. (2011). Ensuring the Data-Rich Future of the Social Sciences. Science, 331(6018), 719–721. DOI: 10.1126/science.1197872
- Lazer, D. (2018). Social science, today. Science, 359(6371), 42–42. DOI: 10.1126/science.aaq0679
- Lazer, D. M. J., Pentland, A., Watts, D. J., Aral, S., Athey, S., Contractor, N., Freelon, D., Gonzalez-Bailon, S., King, G., Margetts, H., Nelson, A., Salganik, M. J., Strohmaier, M., Vespignani, A., & Wagner, C. (2020). Computational social science: Obstacles and opportunities. Science, 369(6507), 1060–1062. DOI: 10.1126/science.aaz8170
- Lazer, D., Pentland, A., Adamic, L., Aral, S., Barabási, A.-L., Brewer, D., Christakis, N., Contractor, N., Fowler, J., Gutmann, M., Jebara, T., King, G., Macy, M., Roy, D., & Alstyne, M. V. (2009). Computational Social Science. Science, 323(5915), 721–723. DOI: 10.1126/science.1167742
- Lepri, B., Staiano, J., Sangokoya, D., Letouzé, E., & Oliver, N. (2017). The Tyranny of Data? The Bright and Dark Sides of Data-Driven Decision-Making for Social Good. In T. Cerquitelli, D. Quercia, & F. Pasquale (Eds.), Transparent Data Mining for Big and Small Data (pp. 3–24). Springer International Publishing. DOI: 10.1007/978-3-319-54024-5_1
- Metzler, K., Kim, D. A., Allum, N., & Denman, A. (2016). Who is doing computational social science? Trends in big data research. us.sagepub.com/sites/default/files/compsocsci.pdf
- Morgan, S. P., & Lynch, S. M. (2001). Success and Future of Demography. Annals of the New York Academy of Sciences, 954(1), 35–51. DOI: 10.1111/j.1749-6632.2001.tb02745.x
- Rosenfeld, M. J., Thomas, R. J., & Hausen, S. (2019). Disintermediating your friends: How online dating in the United States displaces other ways of meeting. Proceedings of the National Academy of Sciences, 116(36), 17753-17758.
- Ruggles, S. (2014). Big Microdata for Population Research. Demography, 51(1), 287–297. DOI: 10.1007/s13524-013-0240-2
- Salganik, M. J. (2019). Bit by bit: Social research in the digital age. Princeton University Press.
- Sobel, B. (2020). A New Common Law of Web Scraping (SSRN Scholarly Paper ID 3581844). Social Science Research Network. DOI: 10.2139/ssrn.3581844
- Venturini, T., & Rogers, R. (2019). “API-Based Research” or How can Digital Sociology and Journalism Studies Learn from the Facebook and Cambridge Analytica Data Breach. Digital Journalism, 7(4), 532–540. DOI: 10.1080/21670811.2019.1591927