Laboratory
Population Dynamics and Sustainable Well-Being
At a Glance
Projects
Publications
Team
Project
Forecasting Individual-Level Mortality
Ugofilippo Basellini, Emilio Zagheni, Monica Alexander (University of Toronto, Canada), Luca Badolato (The Ohio State University, Columbus, USA), Ari Gabriel Decter-Frain (Cornell University, Ithaca, USA), Nicolas Irons (University of Washington, Seattle, USA), Maria Laura Miranda (MPIDR / Federal University of Minas Gerais, Belo Horizonte, Brazil), Erin Walk (MPIDR / Massachusetts Institute of Technology, Cambridge, USA), Elnura Zhalieva (Mohamed bin Zayed University of Artificial Intelligence, Masdar City, Abu Dhabi, United Arab Emirates)
Detailed Description
The prediction of individual-level mortality is a fundamental challenge, with implications for people and societies. Accurate longevity predictions improve life planning, the targeting of high-risk individuals, and the organization of social interventions, policies, and public spending. Demographers and actuaries have been primarily concerned with mortality modeling and prediction at the macro level, leveraging strong regularities in mortality rates over age, sex, space, and time. Apart from clinical settings, predictions of mortality at the individual level have been largely overlooked and have remained a challenging task.
In this project, we look at the individual level: We tackle the overarching challenge of predicting and understanding mortality risk by addressing four interrelated questions. First, we systematically assess limits to micro-level predictability of longevity, considering both traditional and innovative machine-learning methods. Second, we measure how prediction accuracy varies across socioeconomic groups, reflecting the underlying lifespan inequality. Third, we identify key variables to predict mortality accurately and analyze whether they differ across socioeconomic groups. Finally, we compare self-reported survival predictions of individuals with the outputs of statistical models.
In the first stage of the project, we investigate these four issues by using the Health and Retirement Study, one of the most authoritative longitudinal surveys of aging in the United States. So far, we have found that machine-learning and traditional models report comparable predictive accuracy and relatively high discriminative performance, although all models failed to capture the large heterogeneity of lifespans at the individual level. For men, non-Hispanic Blacks and low-educated individuals, predictive accuracy was typically lower than for other socioeconomic groups. People in these groups showed lower accuracy in their subjective predictions of their own lifespan. We further observed small variations in the most important features across groups, with variables related to habits, health history, and finances being relevant predictors. Future work will be directed to the analysis of different contexts and potentially to cross-country comparisons.
Prediction accuracy of individual-level lifespan for different models by gender, race and ethnicity, and education
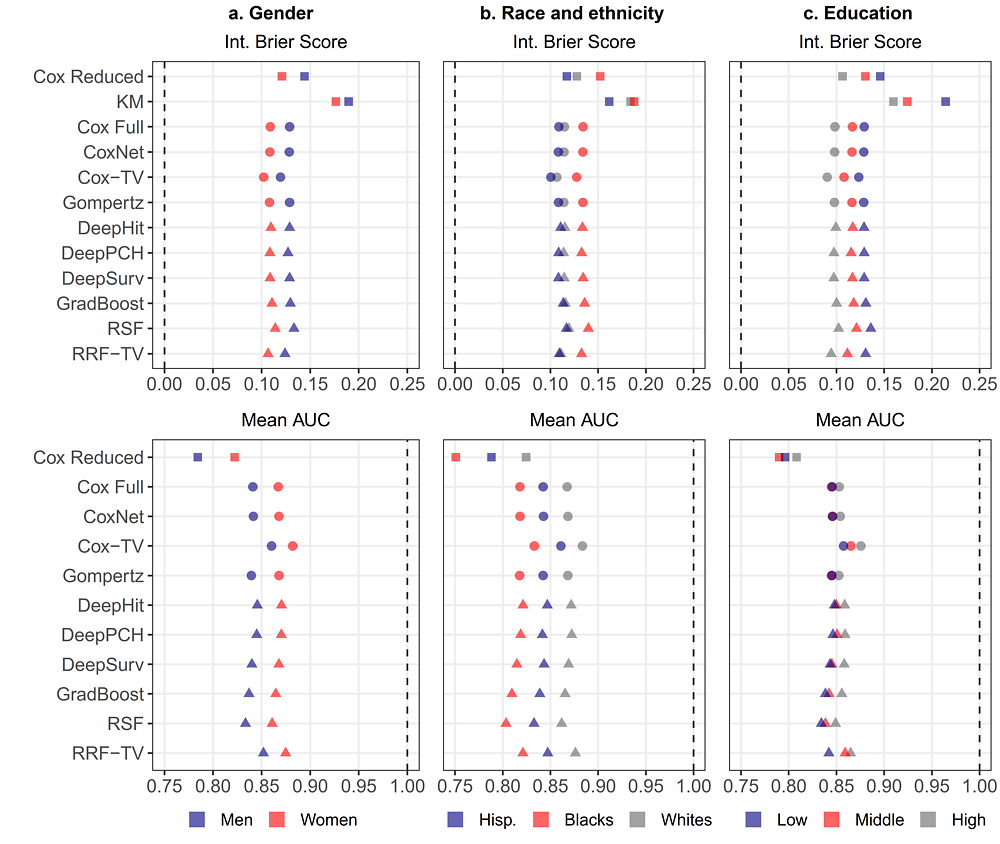
Integrated Brier Score and Mean Area Under the Curve by gender (panel a.), race and ethnicity (panel b.), and education (panel c.). Prediction accuracy across models is worse for Men, non-Hispanic Blacks and low-educated individuals as compared to other socioeconomic groups. © © Badolato et al. (2023)
Aging, Mortality and Longevity, Projections and Forecasting, Statistics and Mathematics
Publications
Badolato, L.; Decter-Frain, A. G.; Irons, N.; Miranda, M. L.; Walk, E.; Zhalieva, E.; Alexander, M.; Basellini, U.; Zagheni, E.:
MPIDR Working Paper WP-2023-008. (2023)
