Laboratory
Migration and Mobility
At a Glance
Projects
Publications
Team
Project
Combining Digital Trace Data and Representative Surveys to Estimate and Predict Migration Stocks and Flows
Emilio Zagheni; in Collaboration with Yuan Hsiao (University of Washington, Seattle, USA), Francesco Rampazzo (University of Oxford, United Kingdom), Lee Fiorio, Jonathan Wakefield (both: University of Washington, Seattle, USA), Jakub Bijak (University of Southampton, United Kingdom), Agnese Vitali (University of Trento, Italy), Monica Alexander (University of Toronto, Canada), Ingmar Weber (Saarland University, Saarbrücken, Germany)
Detailed Description
Reliable and timely estimates of migration flows and stocks are needed to guide policy decisions and improve our understanding of migration processes. However, obtaining timely and fine-grained estimates remains an elusive goal. Often, representative surveys have been used to produce estimates; however, in many contexts such surveys are either not timely enough, not administered regularly, or they cover limited geographic areas. In addition, estimates typically come with high uncertainty because migration is a relatively rare event.
Digital trace data, such as social media geo-located posts, cellphone records, or data from email logs, contain longitudinal, fine-grained information on the time and location of users. These data can be used to improve our understanding of migration processes. However, samples from digital trace data are typically not representative of the general population; statistics taken at face value therefore are biased.
Thus, the crucial question is how to combine the best of both worlds: the representativeness of traditional surveys with the fine-grained geographic and temporal information of digital trace data. We propose a general statistical framework that involves combining digital and survey data for migration estimation by accounting for the bias structure for each data source. If the bias has a structure over time and space that can be statistically modeled, we can combine different sources of data for prediction. Different versions of the same principle are adapted to different contexts.
In a first step, we assume that the estimates from representative surveys are unbiased, whereas the estimates from digital data are biased. We then jointly model the two types of estimates, using a Bayesian hierarchical model that accounts for spatial and temporal effects. The method is used to nowcast internal migration flows in the United States by combining information from the American Community Survey (ACS) and geo-located Twitter data. With a different but related model, the ACS as well as Facebook data for advertisers are used to generate nowcasts of stocks of international migrants in the United States.
In a second step, we account for the possibility that traditional data sources may also be biased. We use a Bayesian hierarchical model that builds on the so-called “Integrated Model of European Migration” in order to combine data from the Labor Force Survey and Facebook data for advertisers in the European context. The aim is to produce an estimate of the number of migrants after assessing the limitations of each data source. In addition, we propose a model to disaggregate the estimates by age and sex. The method can be used to nowcast migration stocks and augment traditional data sources with digital traces, especially when data from surveys or registers lack quality or the needed granularity.
Our models are flexible and can be extended to incorporate multiple sources of data, including cellphone records, administrative reports, survey estimates, and register data. Our approaches can also be extended to other types of demographic estimation beyond migration.
The infographic describes the structure of a framework to estimate migrant stocks using digital traces and survey data
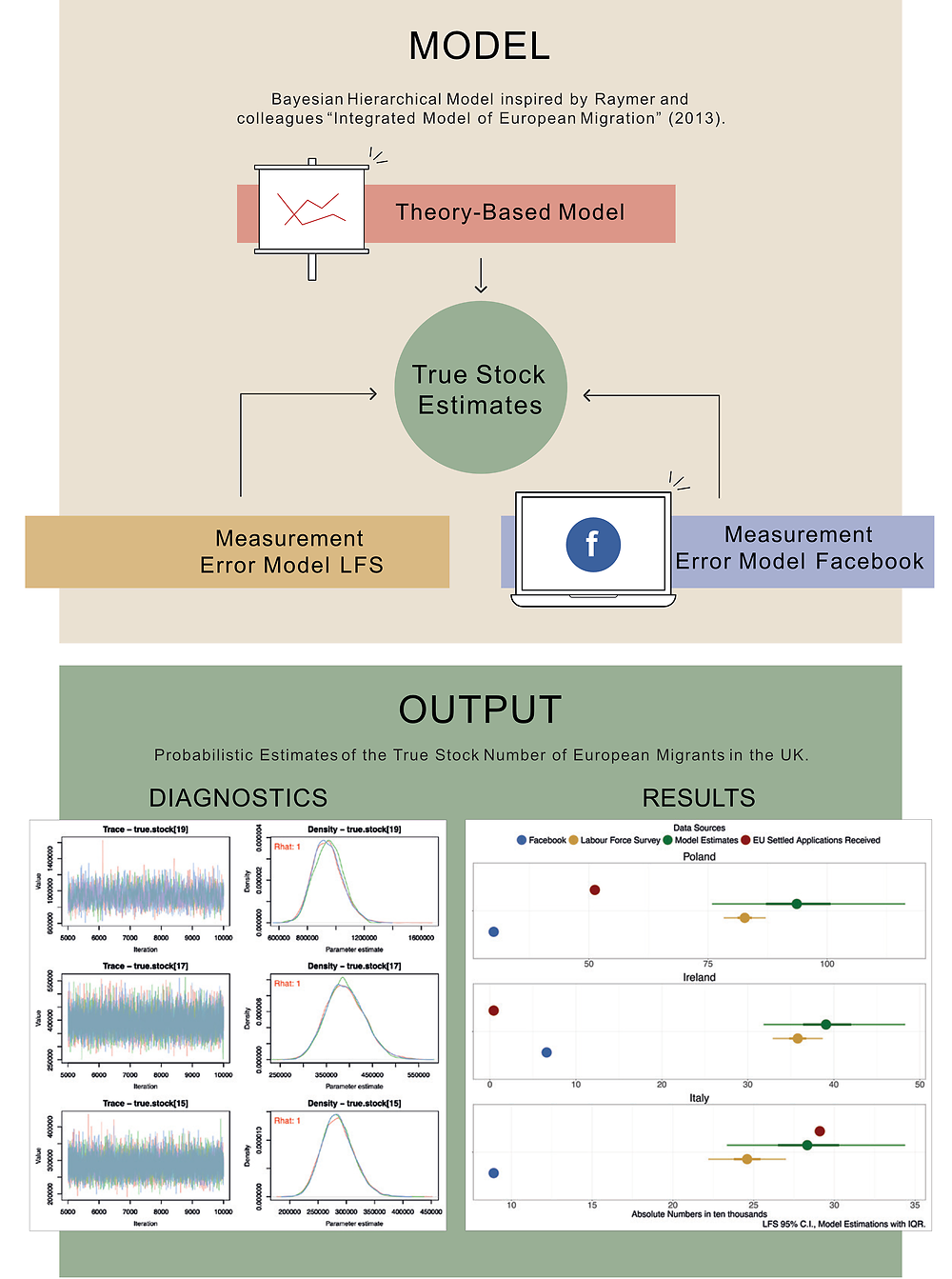
Based on the paper by Francesco Rampazzo, Jakub Bijak, Agnese Vitali, Ingmar Weber, Emilio Zagheni: A Framework for Estimating Migrant Stocks Using Digital Traces and Survey Data: An Application in the United Kingdom. Demography 1 December 2021. © MPIDR
Data and Surveys, Migration
Publications
Rampazzo, F.; Bijak, J.; Vitali, A.; Weber, I. G.; Zagheni, E.:
International Migration Review, 1–22. (2024)

Yildiz, D.; Wiśniowski, A.; Abel, G. J.; Weber, I. G.; Zagheni, E.; Gendronneau, C.; Hoorens, S.:
International Migration Review, 1–29. (2024)

Hsiao, Y.; Fiorio, L.; Wakefield, J.; Zagheni, E.:
Sociological Methods and Research, 1–39. (2023)

Alexander, M. J.; Polimis, K.; Zagheni, E.:
Population Research and Policy Review 41:1, 1–28. (2022)

Rampazzo, F.; Bijak, J.; Vitali, A.; Weber, I. G.; Zagheni, E.:
Demography 85:6, 2193–2218. (2021)

Alexander, M. J.; Polimis, K.; Zagheni, E.:
arXiv e-prints 2003.02895. unpublished. (2020)

Hsiao, Y.; Fiorio, L.; Wakefield, J.; Zagheni, E.:
MPIDR Working Paper WP-2020-019. (2020)
